Powerless Predictions
Sophisticated AI predictions fail to affect policy strategy. Changing that requires taking risks.
Perhaps the earliest instance of far-sighted treatment of promising developments occured before the gates of Troy, where seer Laokoon implored his compatriots not to trust the Greeks’ gift of a wooden horse. Alas, the Trojan decision-making environment was not well set-up: Laokoon was ignored, subsequently devoured by giant serpents, and Troy was sacked.
There is a remarkable disconnect in the world of AI policy. On its one side are sophisticated predictive models that plot out the further trajectory of AI progress; and on its other side is policy work that is remarkably insensitive to the specifics of such predictions. The former side tends to be directionally right about a lot of things, while the latter side has recently failed to score substantial policy wins. It seems fair to ask the groups involved in AI policy: If you’re so smart, why aren’t you winning?
Predictive work on AI progress has grown to occupy a meaningful part of contemporary AI policy discussion. Landmark prediction releases like last month’s AI 2027 and last summer’s Situational Awareness are most immediately visible, but draw on a rich environment of predictive research; take recent work from METR, or the many contributions by Epoch. This often feels like the highest-quality public coming out of the frontier AI policy movement - which counts safety advocates among its founding factions, but is much more heterodox these days, as some have painfully noticed recently.
But for all the praise and interest in landmark works of prediction, they have been remarkably ineffective in affecting or improving policy work conducted by those closest to the predictors - a group of people working on policy that takes frontier AI and its perils and promises seriously.1 Instead, major predictions seem to have largely been successful as external communications providing a synthesis of vibes and predictions to discerning onlookers. This sort of external awareness has had mixed effects at best, and even drawn criticism to predictions on the well-justified suspicion that they make and manifest self-fulfilling prophecies. This kind of effect, in my view, falls short of the effort and talent required by such predictions, and the potential they have. Knowing a little more about what’s about to happen is in itself not particularly helpful. It becomes helpful only when this knowledge is translated into effective policy strategy. This piece is about how that might be done.
What’s Missing?
A lazy contention to the question of ‘why aren’t you winning, then?’ would be that those concerned about AI policy prefer to think and project over doing and advocating. I think this was once true, but by the numbers, there is now a decently large policy environment that does genuine work on genuinely important matters. My contention is rather this: The policy pipeline that runs from in-depth exploration and prediction through to policy planning and political strategy and ends in policy development and advocacy is too narrow in the middle: Between the timeline work outlined above and the policy work done by many organisations, almost no-one is effectively translating takeaways from the former to implications for the latter.
Some policy professionals like to respond to this point by suggesting that this middle work is being done, but it’s done in secret - that policy strategy is too sensitive to be carried out and justified in the open, and so it’s done effectively, but just not visibly. I don’t buy that - first on a cursory look of policy advocacy as it relates to predictions; if that were true, past policy pushes would have better reflected the predictive wisdom. But second also on systematic grounds: Whether it’s regarding predictions or end-state goals, safety advocates in particular have not been shy to publicly volunteer sensitive information at the risk of PR hits and strategic compromise. I struggle to see how this one missing part would have uniquely happened behind closed doors.
Because this middle space meant to directly translate timeline implications into agendas remains underoccupied, a big disconnect arises in what you might consider an AI policy pipeline running from empirical and predictive work through policy strategy and development to on-the-ground advocacy. There is a lack of strategy work translating predictions into political opportunity, and that works to hurt the environment in two ways. First, it renders valuable and interesting work on timelines much less useful than it could be: its most effective applications to policy work remain unused. And second, it in turn renders policy work much less effective:
The result is policy work that misses its quickly moving target because it didn’t bother to consider its trajectory. The 2023 policy pushes for safety everywhere seemed to me an instance of just that: Advocacy focused on the political realities and technical considerations in the here-and-now that failed to price in realistic future trajectories. The policy environment failed to predict increased economic uses that shifted political incentives, leading to the failure of safety summits at the hands of France; failed to predict the increased political polarisation following close alignment with political parties, leading to the repeal of the Biden executive order on AI and the imperilment of the US AISI; failed to price in the escalating imprecision of compute-threshold-based legislation, which contributed to the veto of SB-1047; and failed to appreciate the incentives to provide decent near-term robustness to misuse, which led to exaggerations of near-term harm potentials and subsequent costs to credibility.
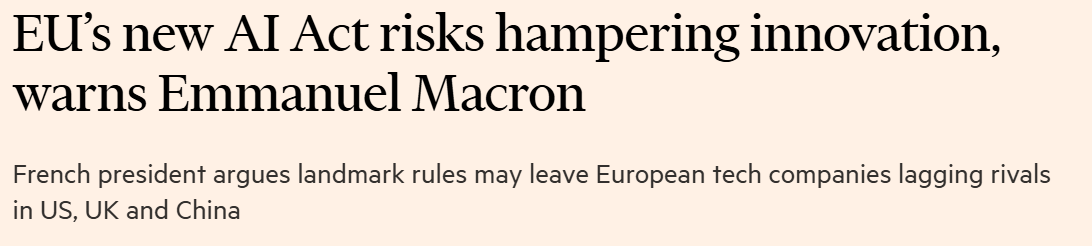
I don’t think these approaches to AI policy reflected or responded well to the predictive wisdom of the time. Had larger parts of the community taken their own predictions of political salience just a little bit more seriously, they could well have anticipated the trends above and avoided their political repercussions. Whatever was executed in 2023 and 2024, it was not a good plan that corresponded well to the predicted realities.
This is not meant to echo the frequent fervent safetyist invocation that ‘there is no plan’, and that going ahead with building advanced AI without such a plan is madness. This strikes me as a heavily reductive view of planning requirements even for complicated problems. Of course there’s no plan for safely deploying superintelligent systems yet: given the many remaining technological, societal and political questions around the nature of that technology, I think such a plan existing would either imply the problem is exceedingly easy, or that the plan will probably fail. I do not think one needs a plan at the same level of fidelity as the most sophisticated predictions - policy will need to be more messy and impromptu. But policy work can still consider these predictions.
Do Timelines Really Matter?
My main sentiment in favour of fixing the timeline-policy-pipeline is that it cashes in on one of the few genuine advantages of the frontier AI policy ecosystem in particular. Most purported advantages do not, or at least no longer, apply: I don’t think safety advocates are all that much smarter or resourceful or intellectually flexible than many other top echelons of policy advocacy. But the deeply community-ingrained penchant for making accurate predictions is a genuinely unique feature, and it slots into a policy environment in which most people are driven to wildly reductive and inaccurate views of the future chiefly guided by intellectual and political idiosyncrasies. In such a setting, seeing clearly can be a substantial point of leverage, and safety advocates are well-positioned to take it. To put it another way: If you were tasked with organising the defense of Troy and incidentally had the - granted, unreliable and sometimes odd - Laokoon in your ranks, would you not ensure the consideration of his inputs?
Many policy professionals look at claims like this and contend: Of course we don’t take complicated predictions seriously – serious policy work is usually made up as one goes, and ten hours of preparative work are usually outweighed by one hour of reactive work. On that view, and with an inbox full of mails and a calendar full of meetings, it’s easy to be Sisyphos rather than Laokoon and just to keep chipping away at it. In its more dramatic version, this sentiment sometimes leads policy types to a dismissive attitude toward Berkeley-coded speculation. I think just as much as the predictors are missing an important reality about the contingency of their policy uptake, this sentiment is an instance of policy professionals missing an importantly unique feature of AI policymaking. In most other policy domains, the sequence and timing of events matters much, much less and leaves much more time to react than in AI – so the general policy professional’s misgivings don’t translate well to this specific instance.
A major reason for that is simply the pace of the technology: Your policy platform can be rendered obsolete or politically unpalatable in just the time you need to develop it. One part of that is technical innovation; if you work to restrict the first deployment of open-source systems past a certain level, but a system like that is already released to little effect, your pitch suffers; if you work to argue for the effectiveness of compute thresholds and a new paradigm or efficiency gain upsets the math behind that, your policy seems dated.
A second reason is high plasticity of the policy environment: Relative to its likely importance, policymakers still know very little about AI, and political platforms have little to say on it. As a result, positions on AI policy are highly volatile: There aren’t any deep commitments or binding party logics that meaningfully constrain the space of possible positions policymakers might take. In many other policy arenas, even a major external event frequently only shifts political positions on the margins; but on AI, external shocks can still actually upset the state of play.
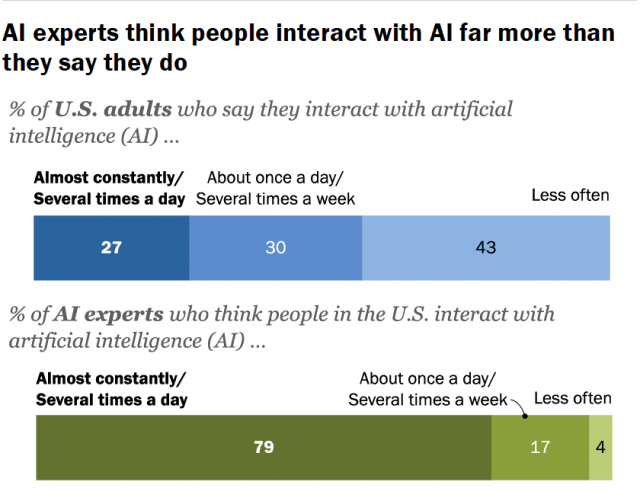
A third major factor are the strong and stormy economic and geopolitical incentives that pull at AI policy in 2025. These incentives render AI policy almost entirely reliant on capitalising on narrow, fleeting policy windows that come up at quite specific instances of the technological trajectory: Surrounding the first cases of major misuse, the first instances of publicly salient labor displacement, etc. It’s hard to make political headway outside these windows, as safety advocates in particular have noticed in recent months: The drive of economic incentive behind the development and the geopolitical motivation to not relent on seeing further progress through reliably outweigh unprompted clever advocacy.
As a result, AI policy specifically is rich in transient political opportunities. But as I have also argued before, these windows do not necessarily provide for one’s policy of choice. They need to be intelligently prepared beforehand and leveraged at once. This, too, implies an outsized importance of informed predictions: The windows open and close fast, and so it is not a viable strategy to simply work on one’s platform and hope to use a window once it occurs. These windows have to be prepared for and leveraged well, and that requires an educated guess on when and how they might occur - plenty of leverage for the far-sighted.
The takeaway from all this is quite simple: I believe that…
…successful AI policy advocacy is mainly a game of using policy windows well
…using these policy windows well comes down to predicting and preparing them well
…the safety community is theoretically well-positioned, but practically ineffective at doing just that.
What Now?
That observation calls for a deeper integration of predictions and advocacy - a widening of the middle section of the pipeline that currently hinders more sophisticated policy strategy. The naive way to do this is to just have the same policy organisations, but have them frequently read pieces and talk to technical experts and attend workshops with hallmark predictors - much as some organisations are doing today. I think this is better than not doing all that, but greatly misunderstands the requirements of acting on predictions still.
I think policy organisations should make bets on much more narrow predictions. One specific AI trajectory will require a very different believable positioning than another: Where labor impacts ‘come first’ on the development trajectory, entirely different alliances and past positionings will be necessary than where catastrophic misuse ‘comes first’. Where heavy nationalisation is in the cards, much different positioning is necessary than where a free market paradigm continues unabated. Each of these require not only that someone at some organisation has thought about them, or even prepared and developed policy proposals to address them; they require that someone has credibility to speak on them. This is only the case when the organisation plausibly cares about whatever issue has become central; and does not appear to merely react and capitalise on a trend.
Taking these differences and their wide-ranging implications for effective policy advocacy seriously means organisations honing in on one of them: To do everything to be in an optimal position if one specific trajectory occurred – to take a bet. That is inherently risky, to an extent that might well be existential to an organisation; if things then shake out a different way, an organisation that took a bet will be rendered nearly obsolete. I think that’s the cost of doing business in this volatile environment, and it’s a fair cost to the potentially much greater impacts on sound policy that could be won.
In some ways, this pitch is a narrower version of my earlier calls for ‘unbundling’ the safety pitch: to have organisations branch out across the spectrum of ideas and narratives to capitalise on windows more effectively. But it’s more narrow and actionable instead. It asks of policy organisations, especially those with the funding and flexibility to do so, of which there are many, to ask themselves a simple question: What prediction on AI progress do we want to bet on? And then it asks them to make a much tougher decision: To uncompromisingly focus most of their policy work on that one specific prediction - on the alliances, the policy development, the political positioning, the hiring and branding that this one prediction will require. An organisation that thinks the labor disruptions story is most plausible would ally and associate much more closely with unions, likely displaced worker groups and the pro-labor elements of respective political parties; and work to develop policy that comprehensively married the frontier (safety) platform to politically opportune measures to smooth labor disruptions.
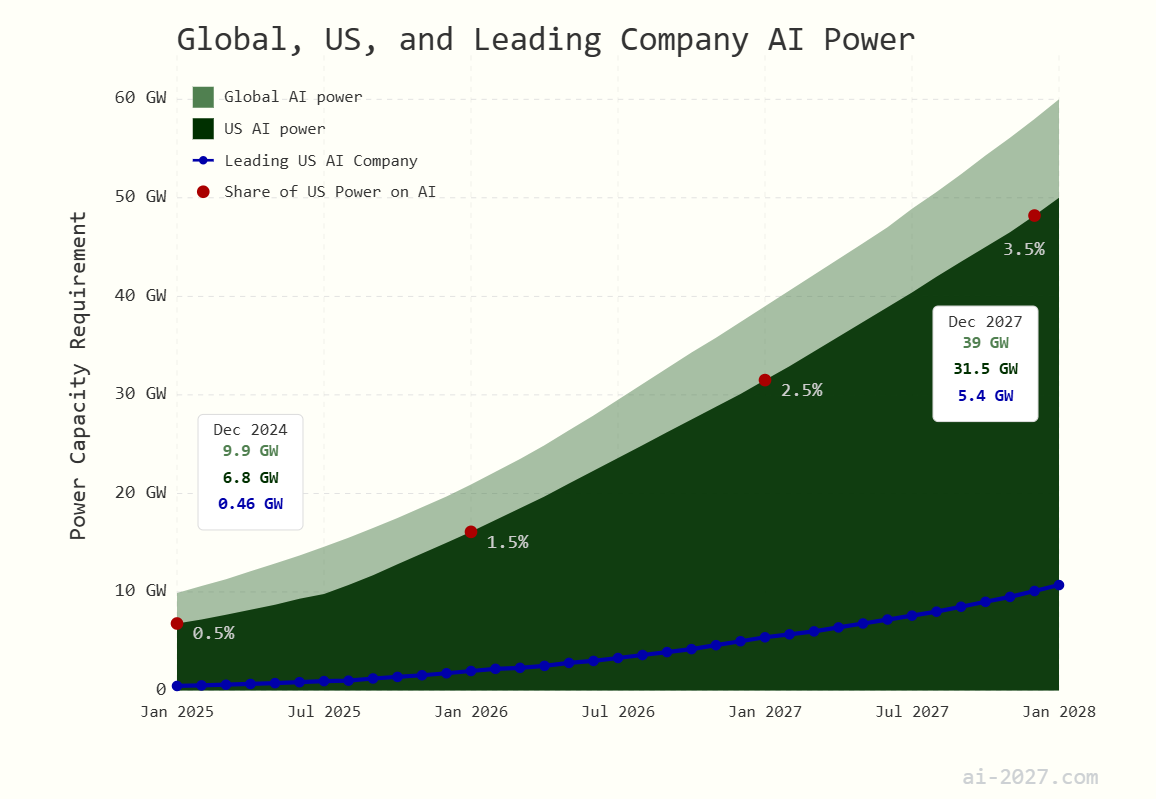
Making that bet dramatically increases the variance on an org-to-org basis - enough, I’d think, to provide a good fighting chance in an increasingly hostile policy environment. The alternative risks a homogenous group of organisations, each with middling credibility and preparation, unable to capitalise on any of the windows that will - predictably! - arise.
No such organisation would be able to lead the policy discussion once it occurred, surrendering ground instead to the incumbents of the area in which the window opens: labor unions and jobs populists with labor disruptions, the national security establishment in questions of defense2 - or as it has already happened, entrenched advocates on fundamental rights on wholesale tech regulation. Bet-hedging organisations risk confinement to the periphery; risk-taking organisations can play for a central role.
Of course the downside is that this strategy is not robust on the organisation level. But I have high hopes that funding, public attention and private ingenuity provide for a sufficiently effective market to ensure robustness on the collective level. In that sense, the often-decried integration and coordination of the frontier AI policy ecosystem is not only a disadvantage - some coordination helps stave off redundancies and overindexing, as long as it avoids drifting into strongly correlated decision-making.
Looking Ahead
It’s tempting to dismiss this proposal by casting it as a belief in mystical abilities of foresight and prophecy. But neither such a belief nor fuzzy appeals to the abilities of ‘superforecasters’ and the like are required to motivate the variance-maximising play. In a tug-of-war of often countervailing incentives, leveraging a policy window is not a matter of perfect insight, but of comparative advantages - all you have to believe to motivate my pitch is that, in the face of messy and chaotic AI politics, serious frontier AI policy organisations are well-equipped to take a clearer look at what lies ahead than most. I’m confident that this plays to these organisations’ strengths - and as long as that is true, there is something to leverage here.
Accurate and sophisticated predictions on AI progress risk becoming at best a vanity exercise and at worst a self-fulfilling prophecy. They could be much more: In a policy environment shaped by who can capitalise on narrow policy windows, predictions can prove enormously valuable. To use them well, organisations have to take decisive bets on these predictions.
Once again, I believe dividing the AI policy landscape into those who take the current trajectories seriously and those don’t provides a reliably more effective lens on the issues than dividing the landscape by one’s attitude to the tradeoff between risks and benefits. At the very least, I’ll keep writing from that assumption - and try to provide work that appeals to everyone that shares my descriptive assessment about the scope of the policy challenge ahead, no matter the normative debate downstream.
In some sense, successes in marrying frontier AI awareness to national security considerations already demonstrate meaningful success in that area. It remains to be seen if ‘AI will be a big security deal’ is sufficiently narrow a perspective to confer deep incumbency advantages to NatSec AI policy organisations, and I have my other grievances with securitisation, but I’d still consider this a decent proof of concept.
Really resonates. In both the US and China, the AI safety movement had an ascendant moment during the 2023 x-risk window, then got eclipsed once hard-nosed state interest math kicked in. Maybe the next step isn’t louder forecasting, but packaging those forecasts in the currency policymakers already trade, like industrial policy, labour disruption, and national-security risk.