The New AI Policy Frontier
Beyond the shortcomings of centralised control and alignment, a new school of thought on AI governance emerges. It still faces tricky politics.
Among the most interesting passages in Thucydides’ telling of Pericles’ Funeral Oration is his invocation of the procedural virtues of democratic enfranchisement: failure to participate renders a citizen not only unambitious, but useless. To treat empowering the citizenry as a mere instrument or hindrance, Pericles argues, misses the point and still imperils the outcome.
For the longest time, AI policy had set its sights on bringing about a carefully conceived end-state of centralised, controlled, well-aligned frontier AI systems. Recent months are bringing about a gradual departure from this consensus. A shift toward more dynamic and decentralised proposals already yields many thoughtful contributions. Perhaps most visible in recent days was the ‘Intelligence Curse’, the most recent entry in the category of microsite-hosted essay collections, which argues in favour of preventing economic and subsequent political disempowerment through distributed AI augmentation. But well beyond landmark essays, a growing frontier of new proposals has begun to emerge.
These proposals are perhaps best understood as tracking part of a greater challenge in the political economy of making advanced AI go well, which is that reigning incentives simultaneously:
(a) favour foregoing extensive and comprehensive developer-side alignment of advanced AI systems, instead prioritising obedience and deployment speed, and
(b) motivate developers to build AI systems that are so capable, centralised and autonomous that they could only be deployed beneficially if they are stringently aligned to societal preferences.
The old-school safetyists’ approach was to provide clever solutions and leverage political incentives to control1 and align these systems, thereby guaranteeing system safety. This pitted them against the incentives motivating (a). This approach has not turned out to be very successful, and also increasingly seems incomplete, as it fails to properly address externalities from economic disruption, disempowerment, and maldistribution.
In that telling of the story, I believe the above-described approaches are best understood as a novel attempt to break free of this dilemma: They aim to accept and endorse a messier reality, where top-down alignment is replaced by a plurality of individualities that maintain freedoms, benefits and safety as a matter of interaction. But in that pursuit, these new approaches run into incentive set (b), which currently pushes toward systems incompatible with decentralisation.
If the new movement to make AI go well is to avoid the fate of the first-generation safetyists, it needs to avoid their mistake of underrating the treacherous political economy it faces. This essay looks at the AI politics of this new frontier.
Political Economy Sank The Safetyists
The initial dominant platform in frontier AI policy - back when it was more synonymous with AI safetyism - centered around controlling and aligning a small suite of powerful AI systems. It first supposed the existence of a select few centrally governed AI systems, developed by powerful AI developers under some degree of governmental oversight, and then suggested acting on that through affecting the proliferation and availability of these agents, as well as through determining how these agents would behave and decide. Success on these terms, people generally imagined, would pave the way toward a bright future, or would at least steer clear of the egregious risks associated with ‘misaligned’ AI, namely advanced AI’s propensity to escape human control and its susceptibility to criminal misuse.
This agenda, and its slight permutations and modifications, has been at the center of a series of policy pushes ranging from international efforts to US-state legislation. Sometimes the content of these policy proposals was more overtly control or alignment-related; sometimes it took aim at the prospect of alignment more indirectly, e.g. through liability or other output desiderata. Either way, these proposals faced powerful incentives, ranging from economic interests of developers in deploying quickly to geopolitical interests in encouraging the international competitiveness of one’s domestic AI industry to predicted military interests in the provision of models that do not refuse deployment to individual customer interests to not be confronted with a chatbot that keeps refusing instructions on unclear ethical grounds. In the face of these stormy incentives, most policy attempts failed.
The recent episodes of models prone to sycophancy and deceptive reward-hacking (the former in the case of a since-retracted update to GPT-4o, the latter in the cases of Sonnet 3.7 and o3) make matters worse: Even where the pitch for centralised alignment is arguably strongest, as it seems to coincide with profit incentives for developers that want to sell reliable models, it can spin into self-defeating pastiche. Bad press and politics for alignment-focused policy follow.2
Simultaneously, messy political and economic realities of advanced AI raise challenges that can no longer be covered by a principally model-alignment-focused approach. Global inequalities caused by differential national deployment, intraeconomic inequalities caused by unequal access to training and inference capability, and job market disruptions through prima facie advisable deployments of productive AI systems are all not easily addressed through provisions on what individual models should and should not do. Against the backdrop of policy failures and the tricky reality of diffusing advanced AI throughout societies, a class of new proposals is arising.
New Proposals On The Horizon
These new proposals take aim at the old agenda’s fundamental assumption of centralised control and deployment. Recognising the practical and political shortcomings of optimising a centralised paradigm, they seek paradigm shifts instead – whether that’s in the form of prioritising AI-augmented human activity (like by Luke Drago & Rudolf Laine), in the form of predistributionary measures to prepare for AI’s economic impacts (like by Saffron Huang & Sam Manning), or in the form of updating institutions and contributions to them in a way that maintains democratic say (like by Séb Krier, Justin Bullock & Sam Hammond) — or in many other forms. This is an admittedly imperfect clustering, and I suspect some authors of some of these approaches would object to my lumping them together. But I think their similarities justify doing so for the purposes of this essay.
These approaches share that they are fundamentally motivated by some perspective of decentralisation: Whereas the previous approaches took a centralised technical and governance paradigm for granted and attempted to steer the content of its governance toward control and alignment, these new approaches put greater stock in addressing the makeup of the centralised paradigm altogether. The direction they all pull in is the empowerment of individuals in the face of the natural centralisation propensity of advanced AI. This is their decisive, shared divergence from much previous AI policy.
There’s a shared suite of technical solutions compatible with that motivation. On the alignment side, it might include a stronger focus on alignment with individual users; on the system design side, it might include a stronger focus on augmenting or otherwise uplifting human ability rather than developing entirely independent agentic systems; and on the model level, it might favour broadly distributed deployment and localised running of efficient systems.
There’s also a policy platform that comes with it: It might consist of encouraging these technical approaches, of incentivising and supporting the provision of economic systems and labor agreements that ensure an enduring place for (uplifted) humans, of ensuring basic access to and ability to navigate uplifting AI capabilities, and of adjusting both sides of individual-government interactions in a way that safeguards and enhances individual’s voices to be effectively heard by institutions and enables the institutions to act on these preferences.
Some question these proposals with regard to their ability to stringently address catastrophic outcomes that gave rise to many earlier AI policy agendas. And in some sense, I agree: None of the above is a ‘plan’ in the same conceptual sense that robust alignment is. I think there’s plenty of promise regardless: both because these proposals are likely to touch on grave and imminent economic and societal pitfalls; and because deploying a more favourable political platform that successfully maintains widespread democratic negotiating power is strong preparation to address future calamity. In fact, many cases of alignment and control going catastrophically wrong feature a breakdown of economic and political institutions somewhere along the way – so safeguarding these institutions seems advisable even on the narrow safety view. So while it might be worth debating if these new proposals are quite as theoretically sound on the edge cases, I consider them promising enough to warrant a deeper look at their own political economy.
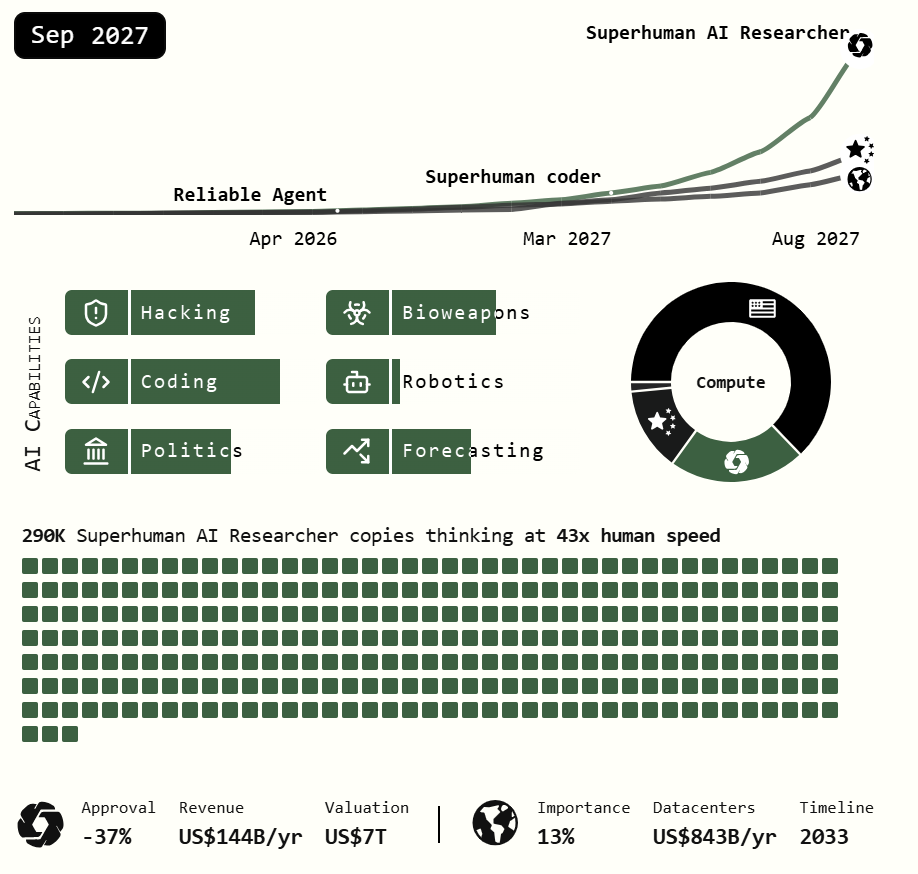
The Challenges They Face
No matter the framing, the politics of getting AI right will not come easy. Despite their many merits, the new proposals still face a similarly treacherous political economy. The threat comes in at least three parts.
Economic Incentives Favour Autonomy
The first part, which falls squarely into the economic part of the incentive set, is that there is strong competitive pressure toward AI workflows that leave humans out entirely. The competitive merit of human augmentation might be extraordinarily short-lived; for just one example, the era of human-augmented chess AIs outperforming pure chess AIs was remarkably short. Even if Marc Andreessen is correct and the elusive skills of venture capitalists will still be required in a post-AGI world, plenty of other tasks will do without human augmentation. And as soon as humans are a burden rather than a boon to an AI workflow, the substantial overhead and maintenance cost of humans becomes a liability to be avoided. Again, much valuable work discusses the exact ramifications of this dynamic. To my mind, the jury is still out whether fast AI progress relegates humans to marginal jobs or makes them uncompetitive for sustenance-level salaries altogether, but neither provides a particularly rosy perspective for maintaining economic power: Economic value would largely come from the contributions made by autonomous AI systems.
The research agendas of major labs suggest they share this economic assessment, as they put an increased focus on creating independent agents with longer and longer task horizons, more and more sophisticated tool integration, and continue to communicate their product midgame not as principally uplift-focused, but as the provision of largely independent systems. A major part of this, I believe, is that they genuinely perceive autonomous agents as the most economically productive deployment of advanced AI capabilities. Part of the incentives that guide this focus surely have to do with moat-building as well; the more sophisticated and well-integrated a developer’s overall platform is, the safer it is from rapidly losing market share to competing models momentarily ahead on cost efficiency. Providing wholesale AI employees, in that logic, is quite the solid moat - because as much as these agent employees will adapt to the logic of their firms, the firms will adapt to their idiosyncratic skill sets and suitabilities, raising the cost for swapping. So for a host of reasons, AI developers will be incentivised to resist this new wave of approaches as well; no longer so much because they don’t want to do too much alignment, but because they don’t want to build the type of product the decentralised approaches require. And every policymaker who is motivated to focus on the economically and strategically optimal deployment might be tempted to follow their lead.
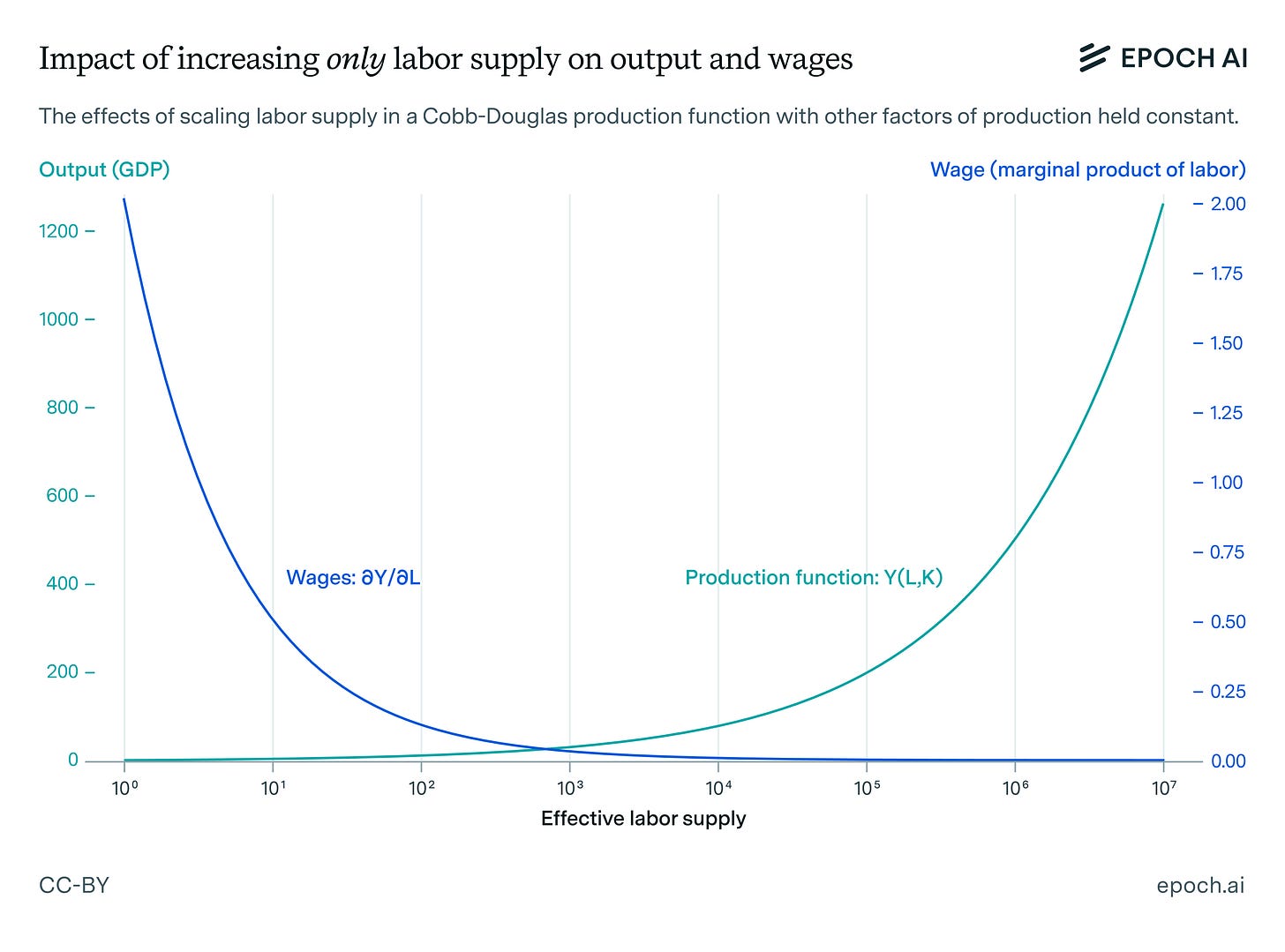
Popular Support Is Not Guaranteed
The broad citizenry to be uplifted is no natural ally of the new approaches, either. On the face of it, a future like the one the ‘Intelligence Curse’ essay series promises seems attractive to the individual: They will be uplifted, not replaced, by the AI system; integrate it in their workflow, depend and interface with their artificial assistant. But this vision has individuals continuously come face-to-face with their increasing obsolescence; it requires a realisation of the scale of AI-powered transformation before accepting the embrace of an AI-empowered paradigm as a solution. Plenty of psychological incentive points squarely the other way, in the direction of sinecure jobs, transfers and a gradual disempowerment that can and will, at times, feel soothing if smoothed by momentary economic relief. As with many other advanced AI policy platforms, new approaches need a lot of good communication before they could hope to gain widespread democratic endorsement.
Policymakers Favour Centralised Control
Lastly, and politically most concerningly, governments are set to lose out on central avenues of enforcement and oversight on the proposed novel paradigms. This is, to the minds of many authors, a feature more than a bug; but it comes with real political costs: It takes away the ability of governments to intervene into the development and deployment in quite the durable way - and once that genie has been let out of a bottle, it would be next to impossible for governments to reestablish control. Anyone who has been around policymakers knows this is a difficult proposition at least.
The history of decentralised technological paradigms tells us that governments mostly let decentralisation happen when they miss out on the outsized importance of technology, and are too late to reintroduce oversight into an already entrenched decentralised system. For just one example, look at the uneasy relationship between the US government and ICANN, where the USG - mostly unsuccessfully - tried to claw back control over the internet after summoning the spirits of multistakeholder governance.
The advocate for decentralised AI alignment faces a much harder political environment still: The default paradigm is already fairly centralised, with the principal alignment and system design for leading models being conducted at the level of a select few, deeply government-involved AI developers. And governments are already quite aware of the importance of AI, and are taking aim at securing its geopolitical benefits through close oversight over model development, over its upstream value chain, and its downstream diffusion. Convincing policymakers to surrender an avenue of control will not be easy.
How They Might Navigate The Cliffs
Taking note of these challenges well in advance can be valuable, as the story of the initial wave of AI policy pushes tells. I think there’s three first steps worth considering in the pursuit of fixing these new approaches’ risky political economy:
Building A Broader Coalition
First, the new approaches still have a decent shot at garnering public support - but as described above, this path goes through some obstacles. To clear them, this needs aggressive leveraging. So far, AI policy often sees an asymmetric mobilisation, where a lot of people don’t care all that much, and the debate is mostly negotiated between marginal interest groups. The perspective provided by the new approaches is in principle a decent basis to change that: Individual empowerment and continued economic relevance should be quite the attractive pitch. This is especially true in comparison to established visions of AI that appeal mostly through system-level effects like growth and innovation that can be hard to understand, and leave questions around the role of any given individual unanswered. The value of the new approaches lies in their direct potential appeal to the affected - it casts them as live players in a technological arms race, as opposed to a soon-to-be-obsolete last generation. That’s a powerful message, but it’s one that relies on two conditions that are not yet met.
The first condition is that the message needs to come from someone who seems trustworthy to the empowered; too much association with the drivers of technological change burdens any political platform with the suspicion of some sort of collusion, whether that’s ultimately framed as an attempt to harvest data or hype technology. To that end, coalition-building around the new proposals is essential; they need to make their way through parties, unions, niches.
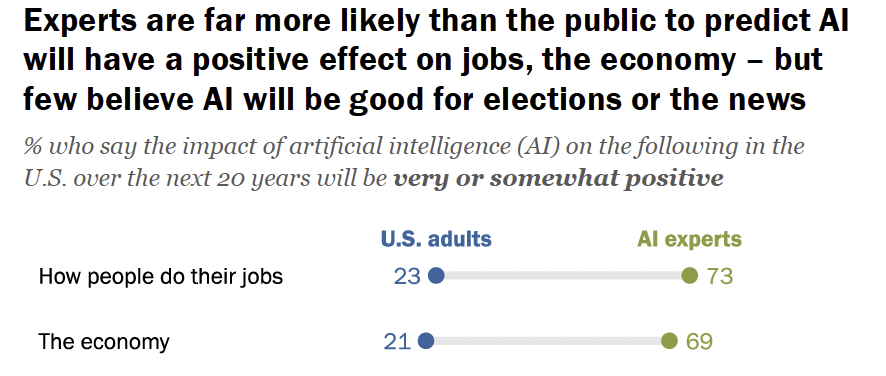
The second condition is that the merits of a decentralised approach cannot simply claim to manifest ‘gradually, then suddenly’. The kind of coalition-building and policymaker persuasion required will probably require iterative instances of success, at the very least in small experimental setups. The policy case for decentralised approaches has to continuously provide hard evidence for its viability – and so the pathway to selling it runs through doing as much as arguing.
This point should not be underestimated based on past evidence. To an extent, original safety policy had it easy. It communicated a discontinuous view of AI progress, to be sure, but a fairly continuous approach to governance by asking for preemptive and central regulatory approaches that could draw on a wealth of precedence from other technology. On the approach-level of abstraction, former policy pushes were eminently prosaic – the new approaches are not, and thus require more trust and proofs of concept.
Separating Societal and Security Desiderata
Second, for new approaches to hold any water in a securitised space, they need to divorce the prosaic economic uses of AI from its national security applications. That’s a tall task, but I feel it’s unavoidable. The closely related incentive set of geopolitical racing and national security deployments politically imperils any proposal that would decentralise: decisionmakers, especially on the security side, will not be inclined to take a risk on decentralisation for broadly civilian or economic political reasons. Getting broad political support right might surmount other policymaker reservations, but not so the more intense geopolitical worries.
What does divorcing these use cases look like? To some extent, this is up to technological happenstance. If and when AI systems and their modes of application branch out further, will the optimal economic tools closely resemble the optimal strategic and military tools? They might not; but I suspect it’s likely they do: I suspect the same AI features that confer economic advantage also play into the strategic dimension, such as the ability to automate workflows and enhance research and development. If the best economic system is the same as the best securitised, the separation is more difficult and heavily favours centralised control. Hence, the new platforms need a very specific response to the question: If we go along with them, do our geopolitical rivals instead go for the centralised approach and outcompete us on the geopolitically essential features? The answer to that question must have a persuasive technological component and an advantageous political framing, or hard security incentives will trump soft societal benefits.
Outlook
I think the new school of decentralised approaches is thoughtful, exciting, and worth considering. It promises a path to addressing some major open questions in advanced AI, and most importantly, maintains humans’ ability to provide their input on the many future open questions that will undoubtedly arise. It’s worth getting the politics on this right - but that will be a challenge.
That is, control not in the technical research sense, but in the broader sense of exerting control over their development, deployment and diffusion.
I know some disagree and might think this is good press for alignment, because it demonstrates the unsolved nature of the challenge. I am very doubtful that onlooking policymakers buy the ‘No True Alignment’ claim that underlies this argument.
nitpick: the Here’s How To Share AI’s Future Wealth essay is linked twice at the start.
through liability - link does not work.